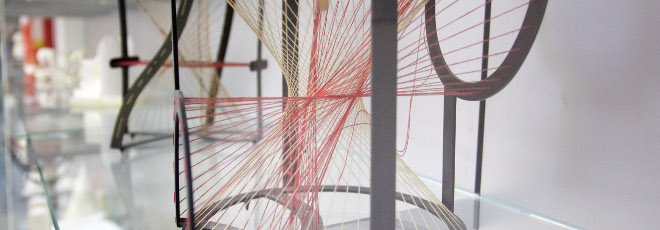
“From Zero-shot Learning to Training-free Generalization”
Lunedì 16 Dicembre 2024, ore 14:30 - Aula 1C150 - Massimiliano Mancini (Università di Trento)
Abstract
Zero-shot learning is the ability of a machine learning model to recognize semantic concepts unseen during training. While over the years this field evolved, a principle remained intact: the key to generalization is grounding the (visual) input to a semantic description of the classes of interest. Nowadays, with the advent of large multimodal models (LMM), the concept of “unseen” is brittle and hard to define. Nevertheless, can this principle still be useful for semantic generalization? In this lecture, we will discuss how this is the case. We will first introduce zero-shot learning and the fundamental ideas for addressing this task. We will then discuss LMMs and their capabilities. Finally, we will see how the principle of grounding visual information to language can be used to re-purpose LMMs to remove assumptions (e.g., in classification) and address tasks beyond the ones they were designed for (e.g., anomaly detection, and compositional recognition) without requiring any training. We will conclude with a discussion of the pros and cons of this paradigm as well as promising future directions.
Bio
Massimiliano Mancini is an ELLIS member and an Assistant Professor at the University of Trento. He completed his Ph.D. at the Sapienza University of Rome, co-advised by Barbara Caputo and Elisa Ricci. During his Ph.D., he was part of the TeV lab at Fondazione Bruno Kessler, the VANDAL lab at the Italian Institute of Technology, and a visiting student at the KTH Royal Institute of Technology. After his Ph.D., he joined the University of Tübingen as a postdoc in the Explainable Machine Learning group led by Zeynep Akata, where he was funded by an Innovation Grant for Science/Life Science of the university. He serves as area chair for major conferences in the field (CVPR, ECCV, NeurIPS, ICRA), where he has also been recognized as an outstanding reviewer (CVPR, ICCV, NeurIPS, ICML) and editor (ICRA). He serves as an associate/area editor for CVIU and TMLR and has organized multiple tutorials and workshops on transfer learning, multimodal learning, and efficient foundation models. His research focuses on efficient transfer learning, continual learning, safety, fairness and compositional reasoning.