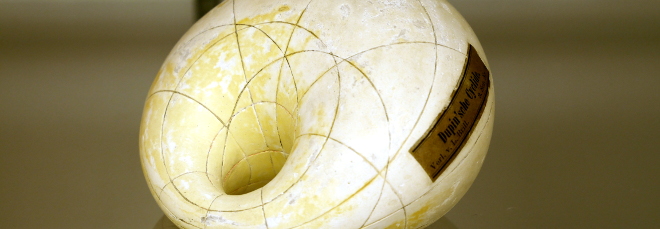
“Approximation by Pseudo-Linear Neural Network Operators and Their Applications”
Tuesday, 26 March 2024, h. 14:00 - Room 2AB40 - Ismail Aslan (Hacettepe University)
Abstract
In this seminar, we discuss the approximations achieved by artificial neural network operators activated by sigmoidal functions, as detailed in [1], and their applications in signal analysis. Notably, Cardaliaguet and Euvrard proposed an approach utilizing neural networks to approximate a function in 1992 [2]. These operators, commonly referred to as neural network operators in the literature, exhibit robust approximation capabilities, even when a function is corrupted by Gaussian noise.
It is well-recognized that nonlinear operators often outperform linear ones in solving real-life problems. Consequently, Bede and his colleagues applied three different types of semi-rings: maximum-product, maximum-minimum, and semi-rings generated by a continuous and monotone generator g (known as the 3rd type pseudo-linear form) in the literature to develop pseudo-linear approximation operators. Their successful outcomes surpassed those achieved by linear methods [3].
While the max-product case is extensively studied in [4], the primary focus of this seminar is to construct the maximum-minimum and 3rd type pseudo-linear neural network operators, along with their Kantorovich forms. We establish convergence results and analyze the rate of approximations. Subsequently, we illustrate our approximations visually. Finally, we apply these operators for signal denoising.
Acknowledgement
This study is supported by The Scientific and Technological Research Council of Türkiye.
References:
[1] Costarelli, D., & Spigler, R. (2013). Approximation results for neural network operators activated by sigmoidal functions. Neural Networks, 44, 101-106.
[2] Cardaliaguet, P., & Euvrard, G. (1992). Approximation of a function and its derivative with a neural network. Neural networks, 5(2), 207-220.
[3] Barnabas, B., Hajime, N., Martina, D., & Antonio, D. N. (2008). Approximation by pseudo-linear operators.
[4] Costarelli, D., & Vinti, G. (2016). Max-product neural network and quasi-interpolation operators activated by sigmoidal functions. Journal of Approximation Theory, 209, 1-22.