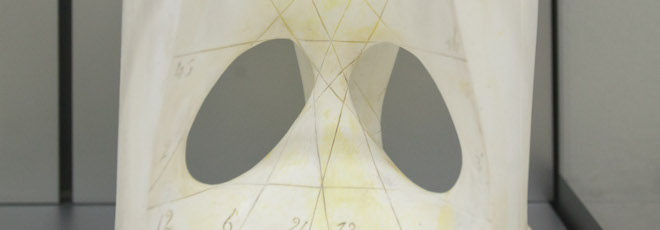
“Detecting anomalies in complex networks”
Venerdì 18 Dicembre 2020, ore 11:30 - Zoom - Gianmarco Bet (Università di Firenze)
Abstract
Recently there has been an increasing interest in the development of statistical techniques and algorithms that exploit the structure of large complex-network data to analyze networks more efficiently. For this talk, I will focus on detection problems. In this context, the goal is to detect the presence of some sort of anomaly in the network, and possibly even identify the nodes/edges responsible. Our work is inspired by the problem of detecting so-called botnets. Examples are fake user profiles in a social network or servers infected by a computer virus on the internet. Typically a botnet represents a potentially malicious anomaly in the network, and thus it is of great practical interest to detect its presence and, when detected, to identify the corresponding vertices. Accordingly, numerous empirical studies have analyzed botnet detection problems and techniques. However, theoretical models and algorithmic guarantees are missing so far. We introduce a simplified model for a botnet, and approach the detection problem from a statistical perspective. More precisely, under the null hypothesis we model the network as a sample from a geometric random graph, whereas under the alternative hypothesis there are a few botnet vertices that ignore the underlying geometry and simply connect to other vertices in an independent fashion. We present two statistical tests to detect the presence of these botnets, and we show that they are asymptotically powerful, i.e., they correctly distinguish the null and the alternative with probability tending to one as the number of vertices increases. We also propose a method to identify the botnet vertices. We will argue, using numerical simulations, that our tests perform well for finite networks, even when the underlying graph model is slightly perturbed. Our work is not limited in scope to botnet detection, and in fact is relevant whenever the nature of the anomaly to be detected is a change in the underlying connection criteria. Based on joint work with Kay Bogerd (TU/e), Rui Pires da Silva Castro (TU/e) and Remco van der Hofstad (TU/e).
Zoom link available at the page Seminars in Probability and Finance page