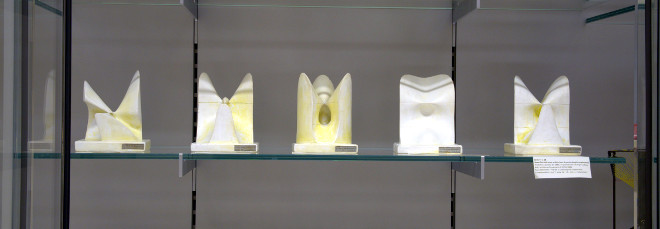
“Gradient-based and Gradient-free Multidisciplinary Optimization”
Wednesday, 6 March 2024, h. 12:00 - Room 2AB40 - Donald R. Jones (Department of Aerospace Engineering, University of Michigan)
Abstract
Modern engineering design problems are often highly complex, involving coupled multi-physics disciplines, time-consuming computer simulations, and nonlinear constraints. Here in the University of Michigan’s “MDO Lab”, we have focused on developing methods for computing the analytical gradients of such coupled systems. Given the gradients, we have been able to solve multidisciplinary optimization (MDO) problems with hundreds of design variables.
However, gradient-based MDO has disadvantages. For example, the computation of analytical derivatives can be difficult and time consuming — and sometimes may not even be possible. In addition, gradient-based MDO is inherently local: different solutions may be found depending upon the starting point, and for some starting points the optimization might not even converge to a feasible point. These considerations have motivated us to look for an alternative gradient-free MDO approach that can handle practical MDO problems of realistic size (e.g., with up to 500 design variables).
In this talk, we describe such a gradient-free MDO method currently under development and show some initial promising results. The method is based on using kriging surrogate models to approximate the objectives and constraints. By exploiting the massive parallel processing capability often available today, we are able to carry out an extensive study of the surrogate models that results in an especially intelligent selection of search points for the next iteration. In a prototype application to a supersonic business jet (41 variables, 3 coupled disciplines, 78 nonlinear constraints), our prototype gradient-free method actually required fewer function evaluations than a standard gradient-based approach.
Short presentation
Donald R. Jones is best known as the inventor of outstanding algorithms as DIRECT and EGO that have changed the game in deterministic global optimization. He served many years as Research Scientist at General Motors and now is a faculty member at the University of Michigan in the Multidisciplinary Design Optimization Laboratory. His ideas and methods have inspired the work of many researchers, generated a huge fallout of literature and has been awarded by General Motors and SIAM. His interests ranges also through response surface methodology and sensitivity analysis and also to the application of multidisciplinary optimization to automotive and aerospace.