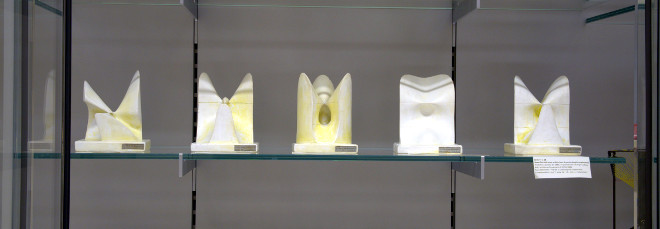
“Modeling sparsity and mixed-binary nonconvex optimization problems”
Lunedì 11 Marzo 2024, ore 10:00 - Aula 2BC30 - Immanuel Bomze (Universität Wien)
Immanuel Bomze and Bo Peng (Universität Wien), joint work with groups from La Sapienza and University of Edinburgh
Abstract
In some ML communities, researchers claim that obtaining local solutions of optimality criteria is often sufficient to provide a meaningful and accurate data model in real-world analytics. However, this is simply incorrect and sometimes dangerously misleading, particularly when it comes to highly structured problems involving non-convexity such as discrete decisions (binary variables). This talk will advocate the necessity of research efforts in the quest for global solutions and strong rigorous bounds for quality guarantees, showcased on one of the nowadays most popular domains – cardinality-constrained models. These models try to achieve fairness, transparency and explainability in AI applications, ranging from Math. Finance/Economics to social and life sciences.
From a computational viewpoint, it may be tempting to replace the zero-norm (number of nonzero variables) with surrogates, for the benefit of tractability. We argue that these relaxations come too early. Instead, we propose to incorporate the true zero-norm into the base model and treat this either by MILP relaxations or else by lifting to tractable conic optimization models. Both in practice and in theory, these have proved to achieve much stronger bounds than the usual LP-based ones, and therefore they may, more reliably and based upon exact arguments, assess the quality of proposals coming from other techniques in a more precise way. With some effort invested in the theory, the resulting models are still scalable and would guarantee computational performance closer to reality and/or optimality.