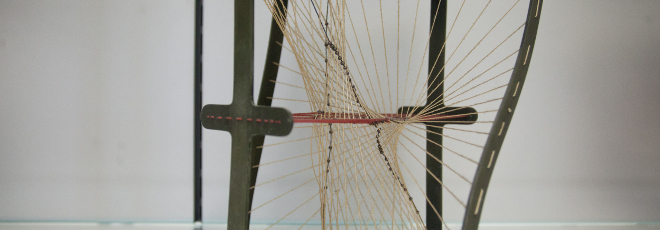
“Probabilistic recursive neural networks for density estimation over generalized random graphs”
Giovedì 19 Dicembre 2019, ore 16:30 - Aula 2AB45 - Edmondo Trentin (DIISM - Università di Siena)
Abstract
Structured data in the form of labeled graphs (with variable order and topology) may be thought of as the outcomes of a random graph generating process, characterized by an underlying probabilistic law. In this talk he will first review the notions of generalized random graph (GRG) and of probability density function (pdf) for GRGs. Then, he will present the main facets of a “universal” learning machine for estimating the unknown pdf underlying an unsupervised sample of GRGs. The machine relies on a maximum likelihood training algorithm, constrained so as to ensure that the resulting model satisfies the axioms of probability. Techniques for preventing the model from degenerate solutions are illustrated, as well as variants of the algorithm suitable to the tasks of graphs classification and graphs clustering. He will report empirical evidence supporting the effectiveness of the approach.