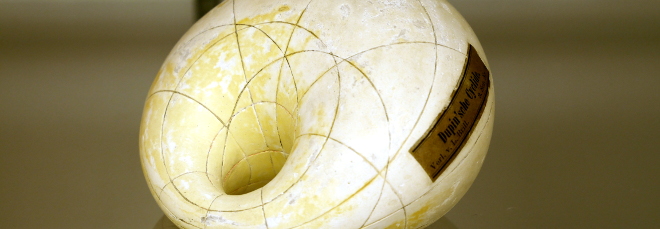
“Wasserstein Generative Models”
Wednesday 13 December 2023 h. 14:30 - Room ASTA - Amna Mohsin (Padova, Dip. Mat.)
Abstract
In this seminar firstly, I will present an introduction about optimal transport. Nowadays optimal transport importance extends to diverse domains, ranging from mathematics and computer science to economics and image processing.
Subsequently, I will talk about the Wasserstein distance, particularly the W1 distance, which is a powerful metric for measuring the dissimilarity between probability distributions and it provides a more stable and meaningful measure than traditional metrics like the Kullback-Leibler divergence. This metric is used in particular within generative models, which are modern deep learning techniques that may be used to generate objects such as images, text, or any other structure. I will introduce these models and explain their application domain and discuss their properties, especially in relation to the limitation in their use of the W1 distance.
Then, I will talk about the main objective of the thesis, which builds upon prior work which introduce a dynamics based method that allows us to obtain very accurate computations of the Wasserstein distance. The objective is to apply this method effectively within generative models to overcome the limitations in the traditional methods used to compute the W1 distance, and how we expect this method to improve the models performances.
The video of the seminar will appear shortly afterwards in this Mediaspace channel.